Boardrooms across the world are buzzing about the implications of artificial intelligence. There is no doubt about it: AI is revolutionizing industries, and the insurance sector is no exception. However, cutting through the noise and zeroing in on specific, achievable AI use cases in insurance can be a bit challenging.
Even after you’ve tracked down some AI in insurance use cases, determining how and where to launch your organization’s artificial intelligence initiative can prove difficult. To help, we’ve compiled valuable information about insurance AI use cases, what it takes to succeed, and what challenges you’ll likely encounter along the way.
Join us as we explore AI insurance use cases to illuminate how you can integrate artificial intelligence technologies into your business model.
In this article we will cover:
Laying the Foundation: The Importance of Quality Data
Before setting your sights on AI insurance use cases, it’s vital for your organization to have a holistic data strategy. You’ve undoubtedly heard the saying, “Garbage in, garbage out.” That proven concept applies to artificial intelligence as well.
Artificial intelligence and machine learning technologies rely on huge amounts of data to learn, draw conclusions, and provide insights. If the data they have access to is incomplete, inaccurate, or just plain unreliable, the insights will be skewed.
While every business leader understands the importance of good data, a shockingly low percentage of organizations have a formal data strategy.
According to a 2022 survey, only 53% of businesses have a formalized strategy for managing their data. This is detrimental to the implementation of any potential AI solution — attempting to implement one without a sound data strategy is like trying to build a skyscraper without a blueprint.
All the organizations highlighted in our insurance AI use cases had mastered the art of data management before rolling out AI. The quality, quantity, and accessibility of their data is a huge reason why their AI initiatives were so successful. Once you’ve got your data in order, you’re ready to dive into the exciting world of AI for insurance organizations.
Do you already understand AI? Ready to dive into specific use cases?
Understanding AI: A Primer for Insurance Leaders
Is your organization ready for AI? The realm of artificial intelligence is brimming with possibilities, but it demands a clear understanding of the technologies, insurance AI use cases, and challenges that lie over the horizon. Here, we break down several types of AI that are particularly relevant to the insurance industry, shedding light on how they can transform your business operations and decision-making processes:
Machine Learning (ML)
At its core, machine learning is about teaching computers to learn from and make decisions based on data. Think of ML technologies much like a student. The more information the machine learning algorithm has access to, the more capable and versatile it becomes. Conversely, if you feed an ML algorithm bad data or starve it of information, it won’t be able to evolve.
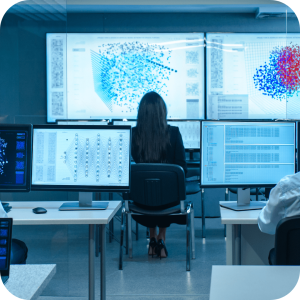
In insurance, this translates to algorithms predicting claim fraud, assessing risks, or event automating underwriting processes. ML can process vast amounts of data, including historical claims, customer interactions, and overall health trends.
During this process, ML can identify patterns that your analysts might overlook. The more data you feed your ML solution, the more accurate and efficient it becomes.
Machine Vision
Machine vision is the process of using computer systems to interpret and understand images, videos, or other visual information. MV technology has already made its way into the manufacturing and autonomous vehicle sectors. As for MV AI insurance use cases, your organization could leverage this technology to analyze medical images, monitor for theft, and conduct quality control.
Machine vision is particularly useful for claims review processes. For example, consider the ability to use machine vision in tandem with drone footage, allowing you to perform crop inspections in the wake of a tornado to estimate the amount and value of any lost crops. The same could be said for evaluating roof damage via satellite imagery following a hurricane.
Natural Language Processing (NLP)
Natural language processing enables machines to understand, interpret, and respond to human language in a way that is both meaningful and useful. A simple example of NLP is chatbots.
Early chatbots could only generate a generic response based on a user’s input, which led to frequent issues. AI-powered chatbots, in contrast, generate dynamic responses, which yield a more human-like conversation.
In the insurance industry, you could use chatbots to handle routine customer queries to keep your support agents free for more dynamic work. When implemented at scale, chatbots have the ability to drastically reduce the workload on support agents and accelerate the delivery of service.
NLP technology is also capable of analyzing customer feedback for insights. Through NLP analysis, you’ll have the ability to gauge customer sentiments toward your services, brand, and offerings.
Predictive Analytics
One of the more exciting AI use cases in insurance is predictive analytics, the most basic form of which is descriptive analytics. The descriptive model helps you understand what happened. Diagnostic analytics is another long-standing analytical model that reveals why something happened.
Predictive analytics moves beyond that and looks to the future. While the concept of predictive analytics isn’t new, human analysts don’t have the capacity to analyze enough data on their own to consistently provide accurate insights. That’s where artificial intelligence and machine learning technology come into play.
Through ML and AI, you can analyze huge amounts of data and obtain accurate predictions about what’s going to happen next. Then, you use this information to guide decision-making processes and push toward organizational goals.
While predictive analytics is exciting, it is not the endgame. When it comes to AI in insurance, use cases include prescriptive analytics. Under the prescriptive model, artificial intelligence technologies analyze massive amounts of data, predict what will happen next, and tell you what you should do to capitalize on those events.
Large Language Models (LLMs)
ChatGPT is the best-known example of LLMs. These robust natural language processing models don’t just understand text- and voice-based language; they can also generate and interact with people in real-time. LLMs are used for language translation, content generation, chatbots, and sentiment analysis.
LLMs are incredibly complicated. The good news is that you don’t have to create your own. Popular platforms, like ChatGPT, offer application programming interfaces, which you can use to integrate an LLM with your other business software.
Understanding these AI types will accelerate your artificial intelligence journey. Each offers unique benefits and can be combined with other technologies to solve specific challenges in your industry. For a more in-depth exploration of artificial intelligence, check out this article from IBM.
Strategy Over Technology
You can’t haphazardly adopt artificial intelligence technology and hope for the best. Implementing any new tech can be expensive and labor-intensive. On top of that, AI is extremely complex, which means you are more likely to encounter some hurdles if you don’t have a sound strategy in place.
On the artificial intelligence journey, AI is the “how,” and your strategy provides the “why.” You can’t determine how to achieve something unless you know what your ultimate goal is first.
After you’ve identified an overarching aim, consider the business impacts of meeting it. If your goal is to solve a problem, such as fraud, identify the implications of leaving the problem unresolved. When organizational decision-makers understand what’s at stake, you’ll have a much easier time garnering support for your AI initiative.
3 AI in Insurance Use Cases
Are you unsure how to leverage AI to solve the problems your organization is facing? Here are three AI use cases in insurance that will serve as inspiration:
Submission Triage
Analyzing new claims represents a major bottleneck for most organizations. Inefficiency during this key process can lead to resource waste and mis-prioritization of submissions. The end result is lost revenue opportunities.
One carrier recently adopted artificial intelligence technologies to automate the process of prioritizing submissions so that underwriters could spend their time on the highest-value claims. By embracing this AI use case, the carrier achieved the following:
- Increased bind rates
- Higher average premiums
- Lower loss ratios
- Accelerated premium growth
The carrier considered expected value and key strategic business levers when implementing its prioritization strategy. Ultimately, the organization achieved a threefold increase in new business growth rate, an increased percentage of higher profit class codes, and higher average premiums.
The artificial intelligence pathway is as follows:
- The triage software takes data from producer performance and submission forms
- It analyzes things like class code performance to predict profitability
- The AI assesses the likelihood that a quote will be bound
- Finally, it determines which submissions warrant immediate underwriter attention
To embrace similar insurance AI use cases, you’ll need historical data on producer bind rates and loss ratios, class code profitability by product, submissions, quotes, and binds.
Download the use case now.
Policy Retention Optimization
Policy retention strongly correlates to long-term business growth. Conversely, high churn rates can erode your momentum and make it hard to hit revenue goals, much less achieve significant growth.
A major carrier used artificial intelligence for retention optimization and achieved astounding results. In this use case, the carrier tapped into the predictive analytics capabilities of AI to determine which members were least likely to renew their policies. They used this data to optimize premiums and improve retention.
Through predictive analytics, the carrier greatly increased retention of profitable policies and boosted underwriter efficiency. They also tapped into relevant data insights to guide rate negotiation processes. Overall, the company improved the retention rate on desirable accounts by more than 50%.
To use AI in a similar fashion, you’ll need historical data on:
- Policy details like premium size, rate, and coverage
- Policy expiration and retention
- Broker information
The carrier mentioned above focused on policies that were unlikely to renew 60–90 days prior to expiration. By doing the same, you can direct your resources toward accounts that may still be persuaded to renew through savvy negotiations.
Download the use case now.
Claims Severity Prediction
Severe claims remain a persistent challenge for insurance carriers, as a handful of major claims can wreck profitability and disrupt the revenue cycle. To combat this, one carrier tapped into historical data to prejudice the future cost of a claim upon receipt of the first notice of loss. The carrier unlocked business value, such as:
- Reduced loss adjustment expenses on low-severity claims
- Faster claims processing
- Improved customer service
- Enhanced high-severity claim outcomes by placing these claims in front of top examiners
One insurer identified that 10% of their claims drove roughly 60% of ultimate losses in bodily injury cases. They also discovered that the lowest 30% of claims only accounted for 2% of total losses.
By analyzing this data, the insurer determined that automating the approval process for low-severity claims would provide major benefits to customer satisfaction and efficiency. And crucially, it would do so without leading to huge losses.
Claims severity prediction uses artificial intelligence to process and analyze historical claims data. The AI then reveals patterns that influence the financial impact of your claims. Machine learning technologies are particularly relevant to this insurance AI use case, as this tech can process structured and unstructured data alike.
Are you ready to apply these AI use cases in insurance to your organization? Connect with UDig, and let’s chat about our bespoke insurance industry solutions.
Download the use case now.
The UDig Difference: Tailoring AI Solutions to Your Needs
UDig has extensive experience in the insurance industry. Our approach mirrors the why-then-how philosophy. We don’t just provide technology; we take the extra step of crafting solutions that align with your unique business challenges and support your growth goals.
Then, we map these needs to specific AI technologies. In doing so, we ensure that the solution addresses the immediate problem and resolves the root cause to propel you forward.
Don’t embark on the insurance AI journey alone. Let the experienced professionals at UDig illuminate the path forward.
Ethical Considerations and Potential Hurdles
After familiarizing yourself with AI use cases in insurance, it is clear that there is plenty of reason to be excited about artificial intelligence. With a strategic approach, you can unlock huge efficiency and cost savings benefits for your organization while also elevating the quality of service you provide.
However, the transition from theoretical understanding to real-world AI insurance use cases is one filled with stumbling blocks and ethical crossroads. Some of the challenges you may encounter along the way include:
Data Privacy and Security
In insurance, the use of AI often involves handling sensitive customer data. If you operate in the healthcare insurance sector, the information you interact with is particularly sensitive and tightly regulated. This raises significant privacy concerns.
Ensuring the security of this data and using it in a manner that respects the privacy of your customers is crucial. This involves adhering to existing data protection laws like HIPAA or the GDPR while also adapting to emerging regulations.
Integrating AI technologies into your workflows expands your digital footprint. In turn, a broader footprint creates new potential avenues for bad actors to exploit. That’s why you must invest in robust cyber security measures and data governance policies that align with these regulations.
Regulatory Compliance
The insurance sector is heavily regulated at both the state and federal levels. AI applications must comply with this complex web of regulations, especially if you use them for underwriting, claims processing, or risk assessment processes.
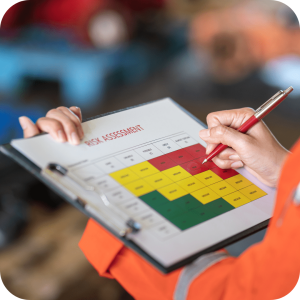
Moreover, you must be mindful of potential biases in risk assessment processes. AI technology excels at performing redundant tasks and controlling for human error, but it is not infallible. You can safeguard your reputation and client data by implementing rigorous quality control processes.
Ethical Conflicts
There’s a growing demand for ethical AI. You must ensure that AI systems do not perpetuate biases or inequities. For instance, AI algorithms in underwriting should not result in unfair pricing or deny coverage based on discriminatory factors, such as gender or sexual orientation.
Remember, too, that AI and machine learning algorithms evolve. Therefore, you must conduct regular audits of your AI systems to check for and correct any biases that may emerge over time.
Transparency and Accountability
According to a 2023 survey, 80% of Americans are concerned that AI will be used for identity theft and other malevolent purposes. Roughly 51% don’t trust AI companies to protect their data.
The bottom line is that the majority of U.S. consumers are worried about the implications of AI. While that shouldn’t dissuade you from embracing these AI insurance use cases, you need to be honest about your plans.
Transparency is the best approach when adopting AI technologies. Let your customers know what you are doing, how it could impact them, and what benefits AI will provide. Also, create an accountability policy and share how you are safeguarding customer data.
Leveraging Insurance AI Use Cases to Stay Ahead
There are numerous exciting AI use cases in insurance. However, the road ahead is undoubtedly filled with challenges and ethical concerns. Finding a partner to help you navigate these uncharted waters is vital.
Enter UDig, a technology solutions provider and consulting firm that combines processes, people, and tech to achieve favorable business outcomes. We’ve successfully deployed AI in numerous industries, including insurance. We know the struggles you face and how AI can help solve them, which means we’re the partner of choice when it’s time to kickstart the insurance AI journey at your firm.
Let’s chat about how AI can drive you forward.